In an age of rapidly advancing technology, humans are fascinated with AI, which is shorthand for artificial intelligence. Many movies and TV shows feature this techno-wizardry, whether it’s AI beings starring in our favorite Marvel movies or the creation of deeply imaginative worlds that depict post-apocalyptic robot takeovers. The idea of deep learning machines is one we often think about in fictional contexts, but at the University of Washington researchers are looking at practical, real-world applications.
Dale Durran, a professor of atmospheric sciences, is on the cutting edge of this search. In particular, he’s using AI to develop a model that will revolutionize our ability to forecast the weather. “We really may be on the threshold of another weather forecasting revolution,” says Durran.
Durran and his team believe that AI can improve the speed and accuracy of simulated weather forecasts on longer time scales. Currently, forecasting for the short term involves using information about today’s weather to forecast the next day’s weather, which is information that can be useful for forecasts up to about two weeks. After that, a single forecast becomes too inaccurate, so additional forecasts are made with small changes to the initial atmospheric conditions and then combined to depict a range of possible weather outcomes. AI can very quickly run significantly more of these additional simulations than current models, representing a greater range of possible weather events over longer time periods.
Durran thinks that “a machine learning approach has the most potential to change how we do sub-seasonal and seasonal forecasts,” which could drastically improve how we prepare for future weather. Weather impacts several aspects of our society, including agriculture, hydropower, flood control and emergency supplies distribution. When we can accurately predict a wetter or drier than average pattern several weeks into the future, we can make necessary adjustments. For example, if heavy rain is forecasted, the water in dams might be let out to produce hydropower and to avoid flooding, knowing that it will be replenished. If conditions will be drier than normal and an area might become susceptible to drought or fires, authorities can bring supplies into those locations to be better prepared.
One way that AI has the potential to revolutionize forecasts on seasonal and sub-seasonal time scales (sub-seasonal is defined as two to eight weeks in the future), is by adding new variables to forecast models. Durran and his team are looking to incorporate satellite imagery to train AI models in ways that are not possible with our current equation-based approach, to create a more holistic representation of the Earth-atmosphere system. One example includes directly placing global cloud patterns observed by satellites into an AI-based model and treating them as a forecast variable. Another is potentially using satellites to look at the vegetation cover in various locations and assessing whether plants are healthy or not, giving forecasters information about local conditions which may also strengthen AI-based models.
To better understand how AI models are different than traditional models, it helps to know how we got to where we are today. In the 1920s, Lewis Richardson was the first to propose using mathematical equations to predict the weather. This was a revolutionary idea but it involved hand calculations and used an elementary mathematical approach that limited the potential for Richardson’s success.
When computers were developed in the 1950s, Jule Charney pioneered the second revolution of weather forecasting using an improved mathematical approach. Durran says one of the first applications for computers was to forecast the weather through equations. This process formed the Numerical Weather Prediction (NWP) model, which underlies current weather predictions today. Now, with the evolution of graphic processor-based computers and using improvements in AI and deep learning, it is possible to replace NWP models.
Using AI to forecast the weather is still in the beginning stages, and Durran and his team continue to test some of its potential applications. He notes that the success they’ve had so far have been astounding, and without many barriers, which gives him confidence in AI’s untapped potential. Durran credits a lot of this success to his former graduate student, Jonathan Weyn, who began looking into this idea. Now, with the help of big players like Nvidia and Microsoft, Durran thinks they will make even bigger strides in using AI to forecast the weather — and this might just be the third wave of the weather revolution.
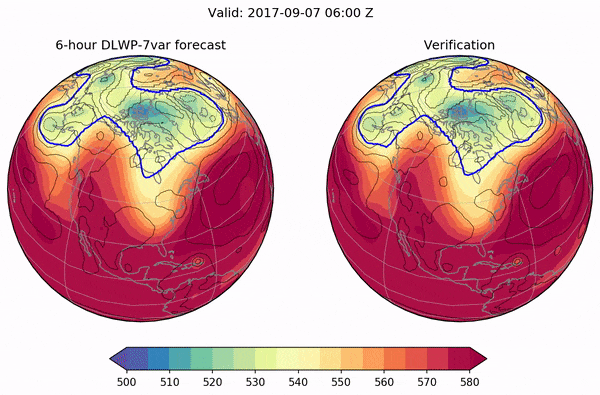